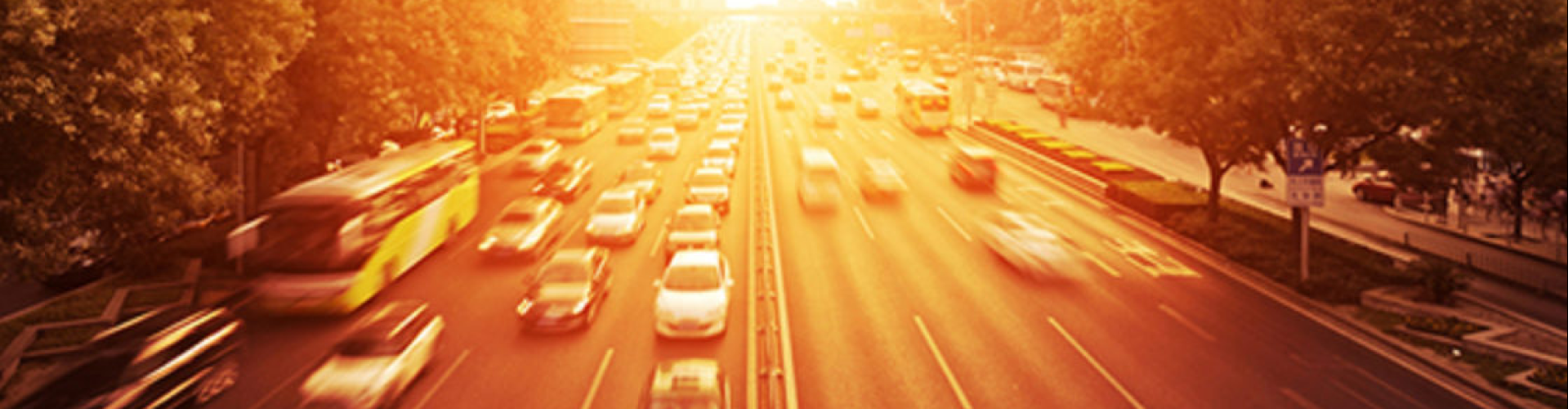
QA Consultants EmTech
QA Consultants Emerging Technologies in Quality Assurance
Learn about QA Consultants’ activities in R&D, Connected Vehicles, Cognitive Autonomous Systems, Artificial Intelligence, Internet of Things, and Blockchain Quality Assurance Services
Focused on the Future
Leading the way
Welcome to the twentieth edition of the EmTech newsletter. In the previous newsletter, we provided insight around the harmonization of Vehicle Regulation (WP.29) and ISO/SAE 21434 standard regarding cybersecurity in Connected and Autonomous Vehicles (CAVs). Now, we will discuss our solution for validation of the HD maps which is essential for the safe operation of self-driving vehicles.
Welcome to our 20th edition of our EmTech Quality Assurance Newsletter
Our emerging technologies quality assurance workstreams
R&D and Grant Projects
Research and development of new technologies that position QAC to become a world leader in quality assurance services.
Connected Vehicles
Testing and Quality Assurance services exclusively developed to provide integration testing services for highly connected vehicles.
Cognitive Autonomous Systems
Fully automation of testing and quality Assurance services exclusively developed for Cognitive Autonomous Systems
Cybersecurity, IoT, AI, and Blockchain
Focus on developing new technologies that utilizes AI to address QA challenges on Cybersecurity, IoT, and Blockchain domains
HD Maps for Autonomous Driving System
Raw map data generated from various sensors might have gaps and other defects. Although map providers are currently working on generating highly accurate maps of public roads with a large coverage, gaps and other defects still exist in the map data and need to be identified. When it comes to autonomous vehicles, occurrences of such defects would impair the localization of the vehicle, and therefore the reliability of the autonomous driving system in the vehicle. Map features need to be validated for large discrepancies. If the differences are such that localization cannot be guaranteed, the autonomous driving system must be notified, thus returning control to the human driver. So, it is necessary to develop a methodology to fully evaluate raw map data and improve its quality (identity and fill gaps).
Testing Challenges
- Limited time to test map data
Current best practices may not offer full map coverage for a limited given time. Therefore, developing an approach that guarantees 100% coverage of map features in a limited time is one of the major challenges. - Limited control on route/road selection
Common best practices suffer from the selection of already validated routes (or repeated testing), which causes lower coverage for a specific time period and repeating already selected test cases. This is due to the random selection of the routes through the implementation of brute force-based algorithms. Thus, it is necessary to have an intelligent route planner to avoid the generation of already verified routes (test cases). - Having repeated test case execution
Variability modeling is essential for defining and managing the commonalities and variabilities. Variability in the different versions of the map data can be considered feature-based variability modeling where the model contains map features such as road, lanes, etc. In common best practices for map validation, map variants are not identified. Thus, there is no mechanism to identify and reuse existing test cases, which leads to wasted resources through the execution of already executed test cases. - The complete automated testing process
Manual testing requires more time and more resources compared to automated testing. Therefore, manual testing should always be minimized to reduce costs. Not all testing processes, including test case generation and execution, are automated in current best practices.
Our Map Test Solution
Our solution introduces intelligence to the current best practice approach for validating HD map data. In this solution, we identify a specific map variant. This means we only test new (or updated) parts of the map data. Therefore, we create an efficient use of testing resources. Given the HD map, desired features, and map database, we identify the variability in data and structure of the map database. The map variant will be obtained by comparing the latest version of the map with the previous versions in the map database. Then, we plan optimized routes (or test cases) for the selected variant based on the test requirements. Utilizing the graph theory concept, we generate the test cases for the map (routes for testing) and the corresponding execution time.
Case Study
We have conducted a case study to validate the proposed approach. In the test, a map is selected for validation. The primary testing requirement is to have a continuous map. In other words, all lanes need to be tested for continuity. In this case study, the goal is the generation of a set of test cases having a minimum number of repeated routes (or redundancy).
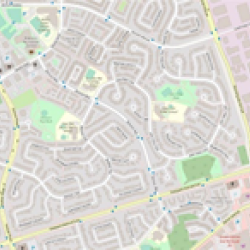
Map in Google Maps format
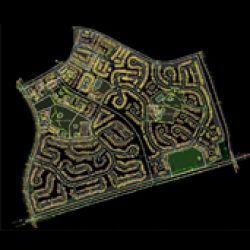
Converted OpenStreet format
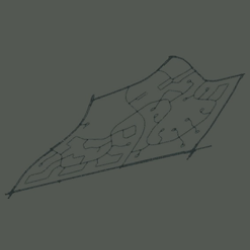
Converted OpenDRIVE format
First simulation
In the first simulation, the original map was the 1st version of the map to be tested. The map had a total length of 63.69KM to be tested. In both approaches, we were able to fully cover the map, however, the testing cost was not the same. Using the current approach, we ended up with 4,478KM of redundancy, thus, over 4,000 extra kilometers. The proposed approach was able to fully cover the map and took around four hours, while for the current approach, we had 299 hours (about 2 weeks). The difference in time and kilometers traveled is enormous.
Second simulation
In the second simulation, we had a different version of the map (some lane segments being removed from the original map, called the second version of the map). We focused on identifying the map variant by partitioning the map and checking each partition against the previous map. The results of implementation for the second version of the map are presented here. Similar to the previous result, the current approach has an elevated level of redundancy and needs a longer time to reach 100% coverage as expected. But the time required for testing the second version of the map using the proposed approach was even further reduced (by 30%) in this implementation. This is due to reusing the current generated test cases for evaluating the unchanged regions.
Validation of the map (reaching the maximum coverage with minimum redundancy)
Parameter | Common Practice | Proposed approach (QAC) |
---|---|---|
Total map coverage | 100% | 100% |
Total length [m] | 63,696.81 | 63,696.81 |
Duplicated distance traversed [m] | 4,478,335.18 | 0 |
Estimated execution time [hours] | 299.36 | 4.68 |
Validation of the 2nd map (reaching the maximum coverage with minimum redundancy)
Parameter | Common Practice | Proposed approach (QAC) |
---|---|---|
Total map coverage | 100% | 100% |
Total length [m] | 62,414.80 | 44,214.00 |
Duplicated distance traversed [m] | 3,521,095.12 | 0 |
Estimated execution time [hours] | 350.44 | 3.29 |
Conclusion
We have developed a deterministic solution for the validation of HD map data that guarantees 100% coverage of map features in a limited time. This approach is scalable and outperforms current best practices in testing HD map data. It is unique in the sense that it can eliminate repeated test validation. Also, in contrast to common best practice, there is no random selection of routes, thus it provides a deterministic solution. In other words, for a given particular input, it always yields the same results.
We were able to identify the map variants and utilize the previously generated test cases to speed up the testing process and save testing resources. The impact of our solution is even more significant when new map updates are delivered for validation. When a map variant is identified, only the selected variant will be used for the validation of the new map. In common best practices, the whole map is usually considered for validation. This includes re-validation of the previously validated parts of the map.
In addition, through developing an intelligent algorithm, we were able to completely remove the repeated test cases. It leads to a significant reduction in testing expenses (time and resources). While using the common practice approach, there is no control on the generation of the repeated test cases due to random selection of the routes.
QA Consultants plans to pursue partners and clients to continually evolve this automated map data testing solution. By doing so, we will encompass the ever-changing datasets that map data companies provide for new and complex solutions.
EmTech
To learn more please visit our EmTech page at https://qaconsultants.com/solutions-and-services/emerging-technologies.